Unveiling the Power of MALDI-TOF MS
Discover the world of MALDI-TOF mass spectrometry (MALDI-TOF MS), a proven technique with ever-expanding applications in species identification.
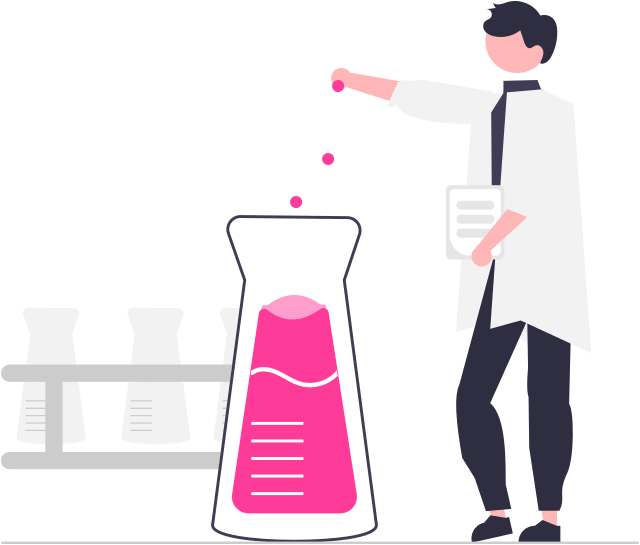
Bacterial Classification Made Simple
BacteriaID takes the complexity out of bacterial classification by automating the identification process. Specifically, it focuses on the automatic classification of toxigenic European ribotypes of Clostridioides difficile into three distinct classes:
- RT027: The most prevalent toxigenic ribotype.
- RT181: An 027-like toxigenic ribotype.
- Other: Any ribotype not belonging to the first two classes.
About Us
BacteriaID is the product of a collaborative effort between two distinguished groups. In the healthcare sector, our solution has been developed in partnership with "Área 4: Microbiología clínica, enfermedades infecciosas y SIDA", a key part of the Instituto de Investigación Sanitaria Gregorio Marañón (IiSGM). This group, deeply involved in clinical and laboratory investigations, contributes to cooperative research centers, and leads infection networks in transplant patients.
Simultaneously, our collaboration extends to the academic realm with the esteemed Department of Signal Theory and Communications (DTSC) at the Universidad Carlos III de Madrid (UC3M). Thanks to its research work, the department boasts a recognized expertise and leadership in projects at both national and European levels. It maintains privileged relationships with numerous national and international companies and research centers operating in these fields.
At BacteriaID, we merge cutting-edge technology with the expertise of healthcare and academic pioneers to offer a seamless and reliable solution for bacterial classification. Join us in exploring the vast potential of MALDI-TOF MS and revolutionize the way we understand and combat bacterial infections.
Our Partners
BacteriaID owes its existence and success to the invaluable collaboration with leading hospitals that have generously shared their MALDI-TOF MS data, contributing to the development and training of our advanced classification model. These visionary healthcare institutions have played a pivotal role in shaping BacteriaID, and we express our deepest gratitude for their unwavering support.
How to Cite Us
The BacteriaID tool
M. Blázquez-Sánchez, A. Guerrero-López, A. Candela, A. Belenguer-Llorens, J. M. Moreno, C. Sevilla-Salcedo, M. Sánchez-Cueto, M. J. Arroyo, N. Calama, A. Martín, V. Gómez-Verdejo, P. M. Olmos, L. Mancera, P. Muñoz, M. Marín, L. Alcalá, D. Rodríguez-Temporal, B. Rodríguez-Sánchez, Rapid, automatic typing of Clostridioides difficile Ribotypes Using MALDI-TOF MS. bioRxiv preprint, 2024. Available at https://doi.org/10.1101/2024.01.24.576988
The Clostridioide difficile dataset
M. Blázquez-Sánchez, A. Guerrero-López, A. Candela, M. Sánchez-Cueto, M. Marín, L. Jiménez-Navarro, N. Calama, A. Martín, L. Alcalá, D. Rodríguez-Temporal, B. Rodríguez-Sánchez, MALDI-TOF spectra of Clostridioides difficile Ribotypes. Zenodo, 2023. Available at https://doi.org/10.5281/zenodo.10370872
Models used by AutoCdiff
A. Belenguer-Llorens, C. Sevilla-Salcedo, M. Desco, M. L. Soto-Montenegro, V. Gómez-Verdejo, A Novel Bayesian Linear Regression Model for the Analysis of Neuroimaging Data. Applied Sciences, 2022, 12(5):2571. Available at https://doi.org/10.3390/app12052571
A. Guerrero-López, C. Sevilla-Salcedo, A. Candela, M. Hernández-García, E. Cercenado, P. M. Olmos, R. Cantón, P. Muñoz, V. Gómez-Verdejo, R. del Campo, B. Rodríguez-Sánchez, Automatic antibiotic resistance prediction in Klebsiella pneumoniae based on MALDI-TOF mass spectra. Engineering Applications of Artificial Intelligence, 2023, 118:105644. Available at https://doi.org/10.1016/j.engappai.2022.105644
A. Guerrero-López, C. Sevilla-Salcedo, V. Gómez-Verdejo, P. M. Olmos, Multi-view hierarchical Variational AutoEncoders with Factor Analysis latent space. arXiv preprint arXiv:2207.09185, 2022. Available at https://arxiv.org/abs/2207.09185